Keyword [ChestX-ray14]
Li Z, Wang C, Han M, et al. Thoracic disease identification and localization with limited supervision[C]//Proceedings of the IEEE Conference on Computer Vision and Pattern Recognition. 2018: 8290-8299.
1. Overview
1.1. Motivation
- building a highly accurate prediction model requires a large number of annotation and finding the site of abnormalities
In this paper, it proposes a method that can work well with a small amount of location annotations
- effectively leverage both class information and limited location annotation
- perform disease identificaion and localization at the same time
- slice the image into patch grids to capture the local information of the disease
1.2. Disease
- large object. Cardiomegaly (心脏扩大), Emphysema (肺气肿), Pneumothorax (气胸)
- small object. Mass (肺部块), Nodule (肺结节)
- Fibrosis (肺纤维化)
- Edema (肺水肿)
- Consolidation (肺实变)
- Atelectasis (肺扩张不全)
- Effusion (肺积液)
- Infiltration (肺部浸润)
- Pneumonia (肺炎)
- Hernia (肺氙)
- Pleural Thickening (肺膜增厚)
1.3. Model

- feature from ResNet-v2 before global pooling layer
- upsample (bilinear interpolation) or downsample (max-pooling) (PxPxc*)
- FCN (PxPxK)
1.4. Loss Function
1.4.1. With Annotated Bounding Box

- i-th image, j-th grid, k-th channel (class)
1.4.2. Without Annotated Bounding Box

1.4.3. Loss for k-th Class
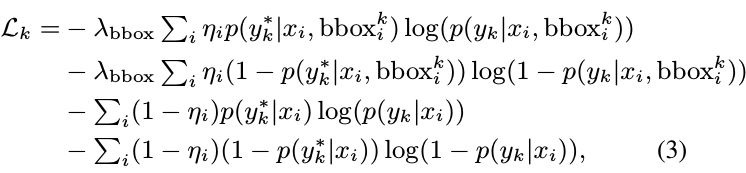
- *. GT
1.4.4. Simplify to
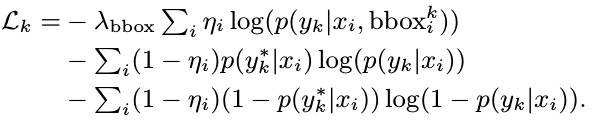
1.4.5. For All Class

2. Experiments
2.1. Details
- patch slice. {12, 16, 20}
- λ_bbox. 5
- normalize the patch scores p and 1-p from [0, 1] to [0.98, 1]
2.2. Dataset
- 112,120 images with 14 disease labels
- 984 bounding boxes for 880 image about 8 disease
- annotated 880 images vs unannotated 111,240 images
- Image. 512x512, [-1, 1]
- no data augmentation
2.3. Classification
- train. 70% annotated + 70% unannotated
- Metric. AUC
